Updated March 29, 2023
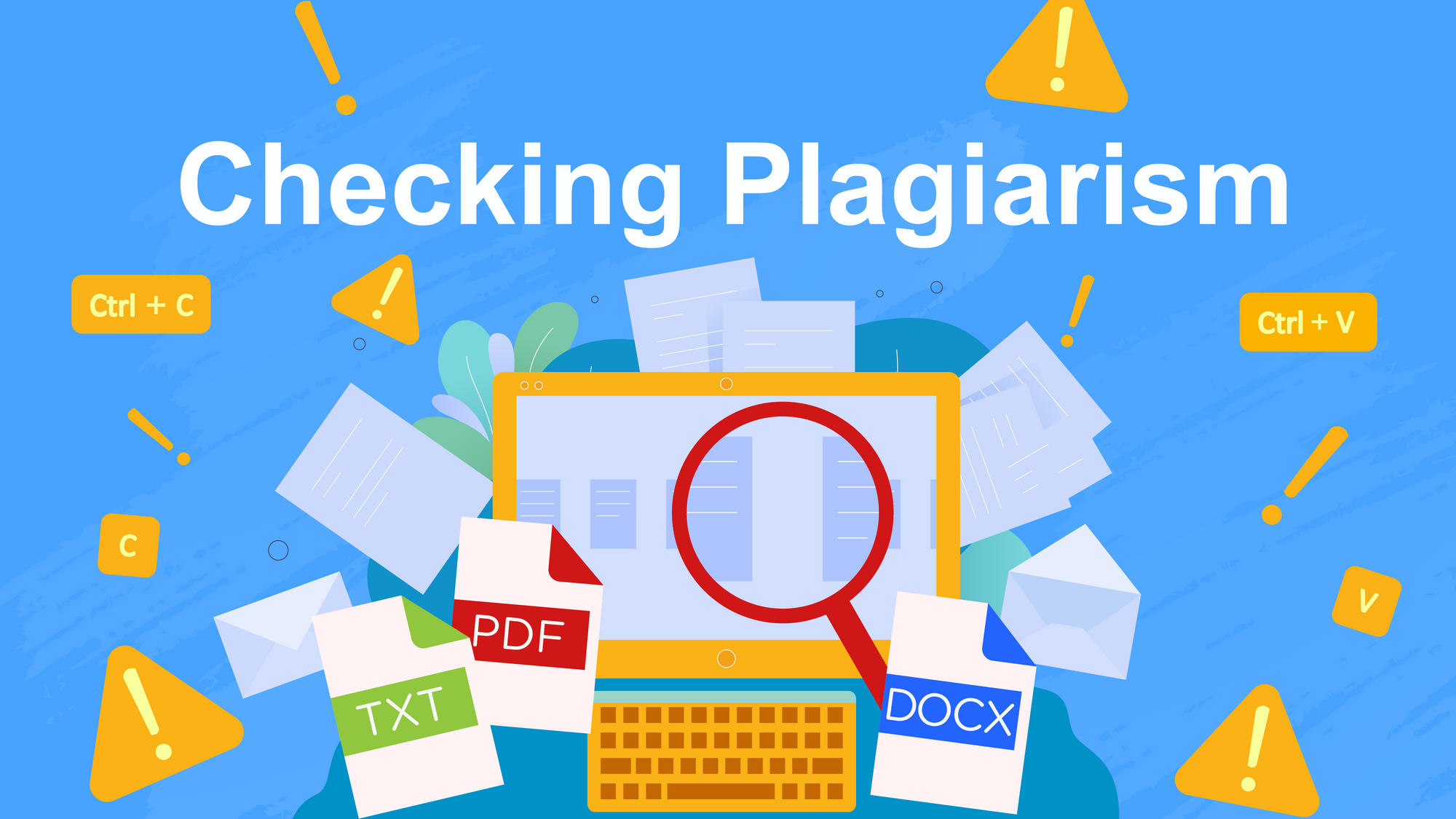
Intellectual dishonesty such as plagiarism comes in many shapes and forms. One of the most obvious and brazen forms of plagiarism is copying someone else's work and passing it off as your own. Sure, it is easy to plagiarize, but these days, with technological advances such as Google, it's also easier to detect plagiarized work. It’s the more sophisticated forms of plagiarism, such as rephrased (but uncited) resources or materials sourced from the analog world, that make plagiarism harder to detect.
Fortunately, for as long as the submitted material is electronic, there’s always a way to determine whether a submission is plagiarized or not. You can also look at readability levels,sentence construction, or even examine specific phrases to compare with other materials previously submitted by other students. There are many plagiarism detectors, but the moodLearning Anti-plagiarism tool (mLaP) is one of the exceptional tools that educators and institutions can use.
mLaP is an innovative tool that automates the routine part of screening for copied parts of submitted documents. The mLaP tool goes through four steps:
- Step 1: Submission
A work or an assignment is submitted in any of the following file formats: txt, pdf, doc, docx, odt, rtf, txt, cpp, java. In Moodle, this is done via the Assignment activity of the course.
- Step 2: Peer Comparison
When a paper is submitted, it undergoes hashing or "fingerprinting" to create its unique identity. The resulting “identity” is compared with other fingerprints from other submissions.
- Step 3a: Internet Search
After Stage 2, the submitted work is compared with online materials that have been indexed by a search engine, regardless of the outcome. Currently, the reference search engine is Bing.
- Step 3b: Network Search
To enhance the search process, an additional layer of search is done with the moodLearning Native Search (mLaPNS) indexing particular networks or domains. This feature also allows individuals or organizations outside of any learning management system to upload documents that can be used as a reference or added to the database. See also use-cases-of-mlap-native-search
- Step 4: Results
Finally, the results from comparisons done in Stages 2 - 3 are consolidated and displayed as percentages of similarities. Such results should enable the evaluator of materials to determine whether plagiarism has occurred.
As can be seen from the steps above, you can compare students’ submitted work with the materials submitted by their fellow students as well as compare it with the vast amount of materials available online. Somehow, the evaluators or the teachers must have the confidence in their own ability to flag plagiarized work.
Such confidence (or lack of it) is only probabilistic. At what percentage of the whole document would prove conclusive that it is plagiarized? 10%, 30%, 50%, 70%? The higher the percentage of similarities between the submitted document and other previously submitted documents (whether online or those submitted by their fellow students), the stronger is the teacher’s confidence that the submission is plagiarized. It’s the lower numbers that may prove to be rather tricky – and it’s never an exact science.
Like any probabilistic tools, plagiarism-detection apps are bound to yield either false positive or negative results. It can either tell us there’s a likelihood of plagiarism where there’s none, or tell us there’s no plagiarism when there’s one in fact. (See, for instance, this piece from Inside Higher Ed)
One of the key advantages of using mLaP is that it automates the routine part of screening for copied parts of submitted documents, saving teachers and raters time and effort. This allows them to focus on more important aspects of their work, such as engaging with students and providing constructive feedback.
Another positive aspect of mLap is that it can help create a culture of academic integrity by providing students with clear and objective feedback on their work. Processes or systems can be put in place where students do not have to plagiarize any material. Do you see gradual progress between this version and the later versions? Or do you see a sudden improvement in a student’s “eloquence” that may need a second look at his work? By using mLaP to screen for plagiarism, teachers and raters can ensure that students understand the importance of originality and attribution and encourage them to develop good habits that will serve them well throughout their academic and professional careers.
This is the kind of context where plagiarism detection is properly put. The moodLearning anti-plagiarism (mLaP) tool offers several advantages which is explained in more detail here:
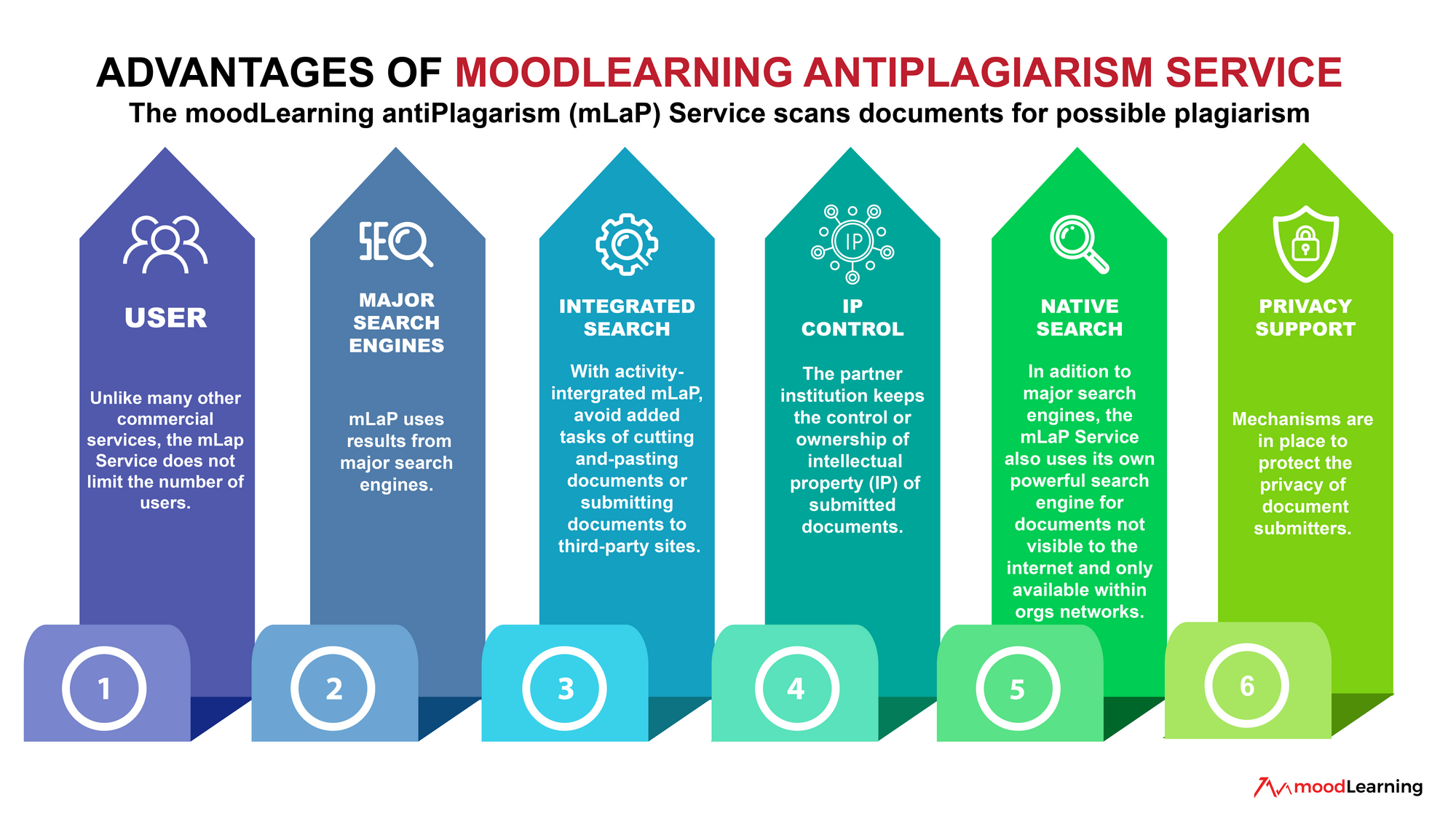
- Unlimited number of users. Unlike many other commercial services, the mLaP Service does not limit the number of users. It comes bundled with the service contract with moodLearning.
- Combined power of major search engines. Using the results from major search engines like Bing, the mLaP Service searches the Internet for similar documents to be compared with student submitted documents, to check for possible plagiarism.
- Integrated search. The mLaP Service is embedded right in the settings of Assignment submission, thus avoiding the added task of submitting or cutting-and-pasting student papers in third-party online portals.
- Intellectual Property (IP) Control. The partner institution keeps the control or ownership of IP of submitted documents from its constituents. These files do not leave the institution's installation.
- Native search engine. In addition to the combined power of major search engines, the mLaP Service has its own powerful network search engine (moodLearning Search) that can be used to index contents found within a particular network or domain.
- Privacy support. The mLaP service provides privacy support to submitters of documents.To guarantee the safety and security of personal data, mLaP uses SSL (Secure Sockets Layer) encryption to protect student data during transmission from the user's computer to the mLaP server and stores all submissions in a secure data center that is monitored 24/7. Compared with the leading plagiarism scanner in the market, mLaP cannot be far behind either.
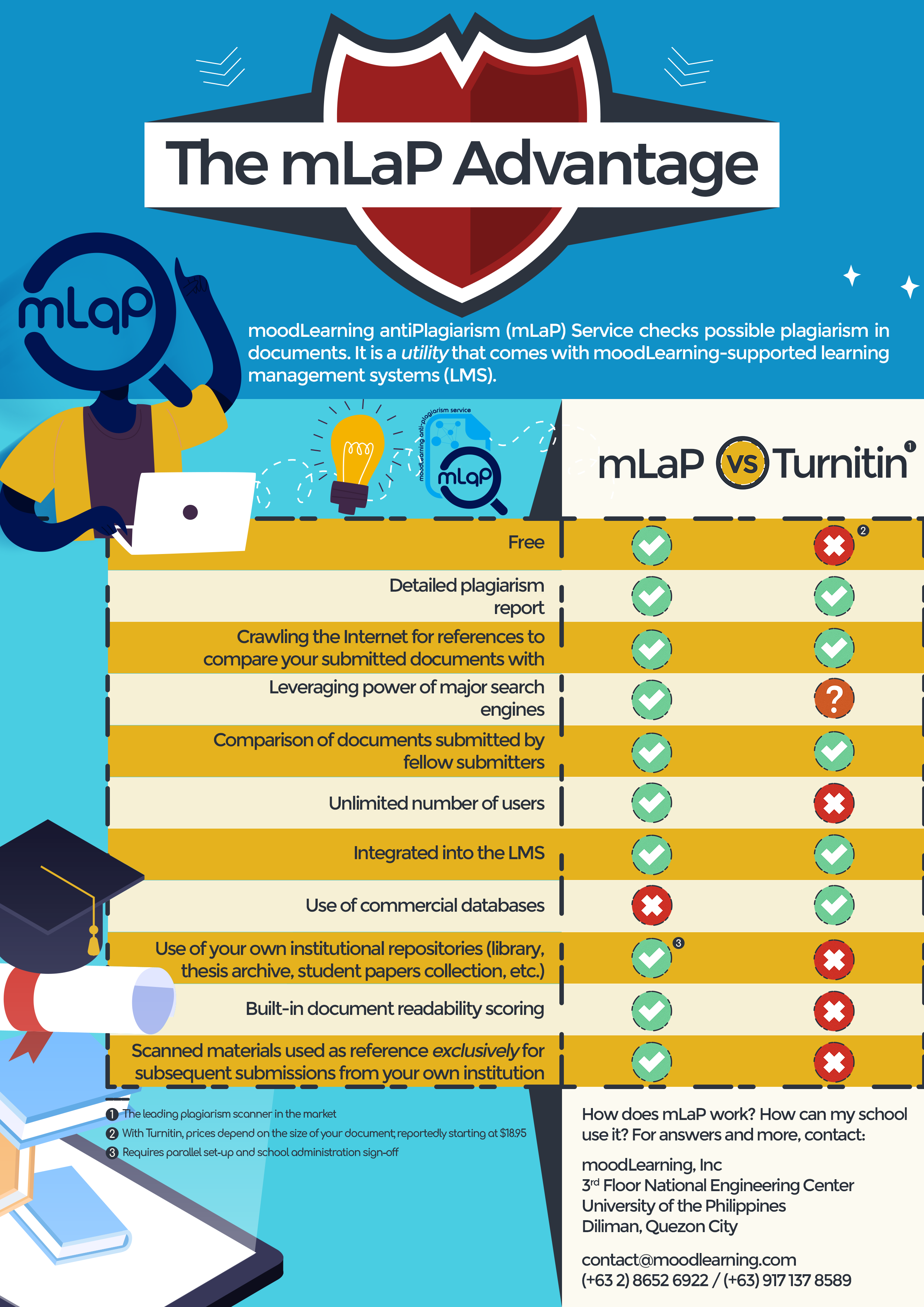
In conclusion, the moodLearning anti-plagiarism (mLap) tool is a powerful and valuable resource for educators, students, and researchers alike. By leveraging its capabilities to identify instances of plagiarism and promote academic integrity, we can create a more honest, ethical, and productive learning environment for everyone.
Do you think an anti-plagiarism service like mLaP needs to be integrated into your own learning management system? Talk to us at moodLearning (contact@moodlearning.com).